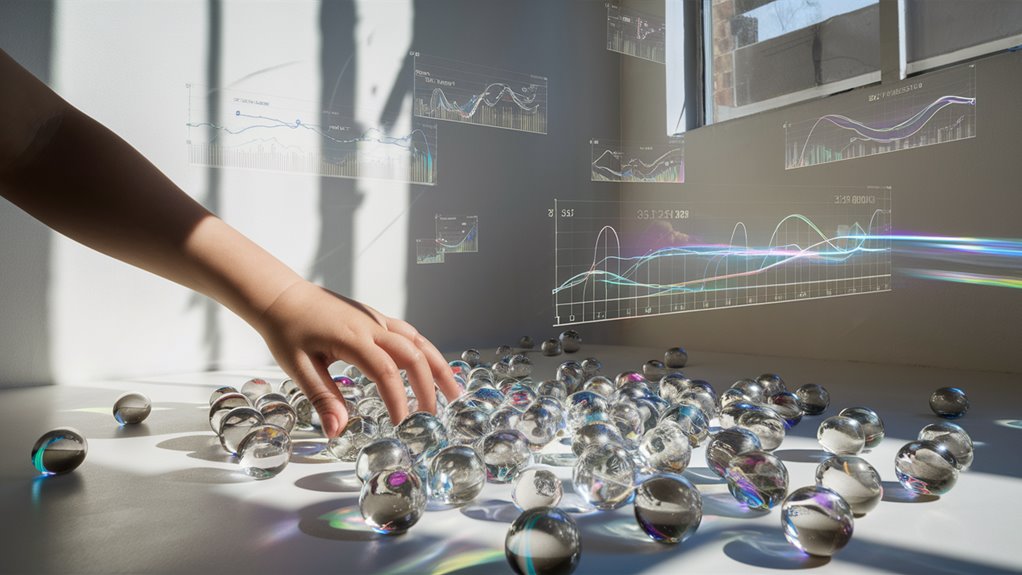
The Art of Data-Driven Intuition: Mastering Analytical Synthesis
*Data analysis* and *creative exploration* form a powerful partnership in modern problem-solving. By integrating *structured analytical methods* with *intuitive pattern recognition*, professionals can unlock deeper insights from complex datasets.
The Foundation of Analytical Synthesis
*Statistical rigor* combined with *creative thinking* creates a robust framework for discovery. The optimal approach allocates *70% systematic analysis* and *30% experimental exploration*, establishing a balance between methodical investigation and innovative thinking.
Leveraging Modern Tools for Enhanced Insights
*Advanced visualization platforms* and *machine learning algorithms* amplify this synthetic approach. These tools reveal *hidden patterns* and *correlations* that traditional numerical analysis might miss, enabling a more comprehensive understanding of complex data relationships.
Frequently Asked Questions
Q: How does analytical synthesis improve decision-making?
A: It combines data-driven insights with intuitive understanding, leading to more balanced and informed decisions.
Q: What role does machine learning play in data synthesis?
A: Machine learning algorithms identify complex patterns and relationships that might be invisible to traditional analysis methods.
Q: Why is the 70/30 split between systematic and experimental approaches effective?
A: This ratio maintains analytical rigor while allowing sufficient space for creative discovery and unexpected insights.
Q: How can visualization tools enhance data synthesis?
A: Visual representations make complex data patterns more accessible and help identify relationships that might be missed in raw numerical data.
Q: What skills are needed to master analytical synthesis?
A: Professionals need strong statistical knowledge, creative thinking abilities, and familiarity with modern data visualization tools.
Key Benefits
- *Enhanced pattern recognition*
- *Deeper insights into complex datasets*
- *Balanced decision-making framework*
- *Improved problem-solving capabilities*
- *More comprehensive understanding of data relationships*
The Dance of Data
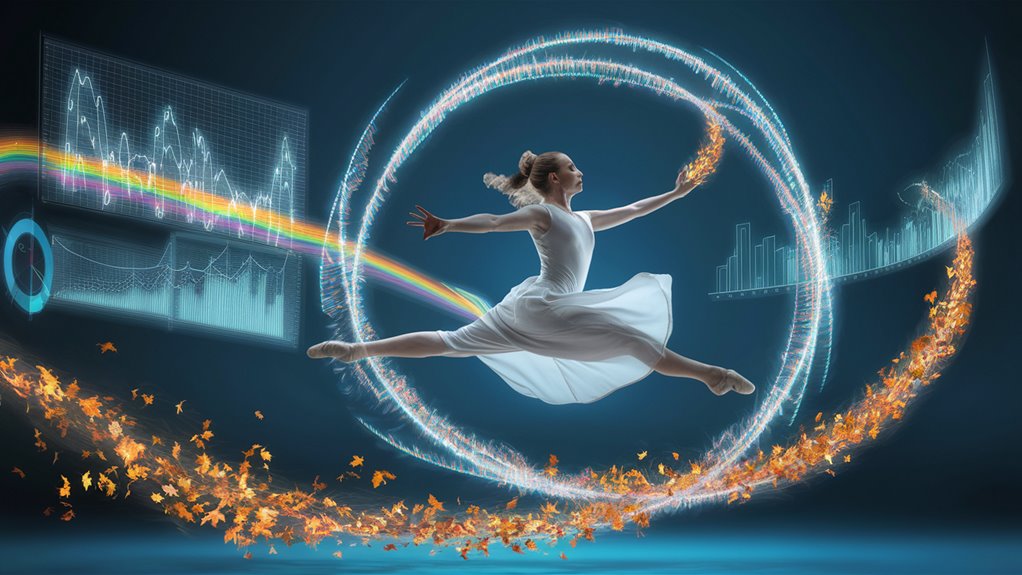
The Dance of Data: Understanding Modern Analytics
The Choreography of Information Analysis
*Data analytics* has evolved into a sophisticated performance where *numbers and insights* move in harmony to reveal profound business intelligence.
Like a carefully orchestrated dance, modern *data analysis* combines technical precision with creative interpretation to unlock valuable patterns within complex information systems.
Key Elements of Data Movement
*Statistical analysis* flows through multiple dimensions, with *correlation patterns* and *regression models* working in synchronized motion. These analytical elements create a dynamic framework where:
- *Data patterns* emerge organically through systematic observation
- *Statistical relationships* reveal themselves through careful analysis
- *Outlier detection* provides crucial insights into anomalies
- *Trend analysis* maps the directional flow of information
The Art and Science of Pattern Recognition
*Data interpretation* requires both structured methodology and intuitive understanding.
Successful analysts master the balance between rigid statistical frameworks and flexible pattern recognition, allowing them to:
- Identify meaningful relationships between variables
- Adapt analytical approaches based on emerging patterns
- Maintain mathematical rigor while exploring new connections
- Transform raw data into actionable insights
FAQ: Understanding Data Analytics
Q: What’s the most important skill in data analysis?
A: *Pattern recognition* combined with statistical expertise is crucial for effective data analysis.
Q: How do you identify meaningful data relationships?
A: Through systematic observation of *correlation patterns* and statistical testing.
Q: What role does flexibility play in data analysis?
A: Flexibility allows analysts to adapt methods and discover unexpected insights while maintaining analytical rigor.
Q: How can businesses benefit from data pattern recognition?
A: By identifying trends and relationships that inform strategic decision-making and operational improvements.
Q: What tools are essential for modern data analysis?
A: Statistical software, visualization tools, and programming languages that support both structured and exploratory analysis.
Mastering the Rhythm of Information
Success in *data analytics* comes from maintaining perfect timing between structured analysis and creative exploration.
This balanced approach ensures that insights emerge naturally while adhering to robust statistical principles.
The result is a powerful analytical framework that transforms complex data into clear, actionable intelligence.
Playing With Patterns
Playing With Patterns: A Data Analysis Approach
Understanding Pattern Recognition in Data
*Pattern recognition* has emerged as a powerful tool in modern *data analysis*.
By treating complex datasets as opportunities for discovery rather than mere collections of numbers, analysts can unlock deeper insights and meaningful correlations.
*Visual pattern recognition* serves as a fundamental approach to understanding intricate relationships within data structures.
The Power of Exploratory Analysis
*Exploratory data analysis* combines *traditional statistical methods* with innovative visualization techniques. This dual approach enables analysts to:
- *Identify hidden trends* through visual representation
- *Discover unexpected correlations* across diverse datasets
- *Develop intuitive understanding* of complex relationships
Advanced Pattern Detection Strategies
*Data visualization* techniques transform raw information into actionable insights.
By implementing *dynamic pattern recognition*, analysts can:
- Map relationships between seemingly unrelated variables
- Detect anomalies that might indicate significant trends
- Create predictive models based on historical patterns
Frequently Asked Questions
Q1: What’re the key benefits of pattern-based data analysis?
A: Pattern-based analysis enhances insight discovery, improves predictive modeling, and facilitates better decision-making through visual recognition of trends.
Q2: How does visual pattern recognition differ from traditional statistical analysis?
A: Visual pattern recognition offers intuitive understanding through graphical representation, while traditional statistics provide numerical validation of findings.
Q3: What tools are essential for pattern-based data analysis?
A: Key tools include data visualization software, statistical analysis platforms, and machine learning algorithms designed for pattern recognition.
Q4: How can organizations implement pattern recognition effectively?
A: Organizations should invest in proper tools, train analysts in visual analytics, and establish clear protocols for pattern validation and verification.
Q5: What’re common challenges in pattern-based analysis?
A: Common challenges include data noise, false correlations, and the need to validate apparent patterns through rigorous statistical testing.
From Numbers to Narratives
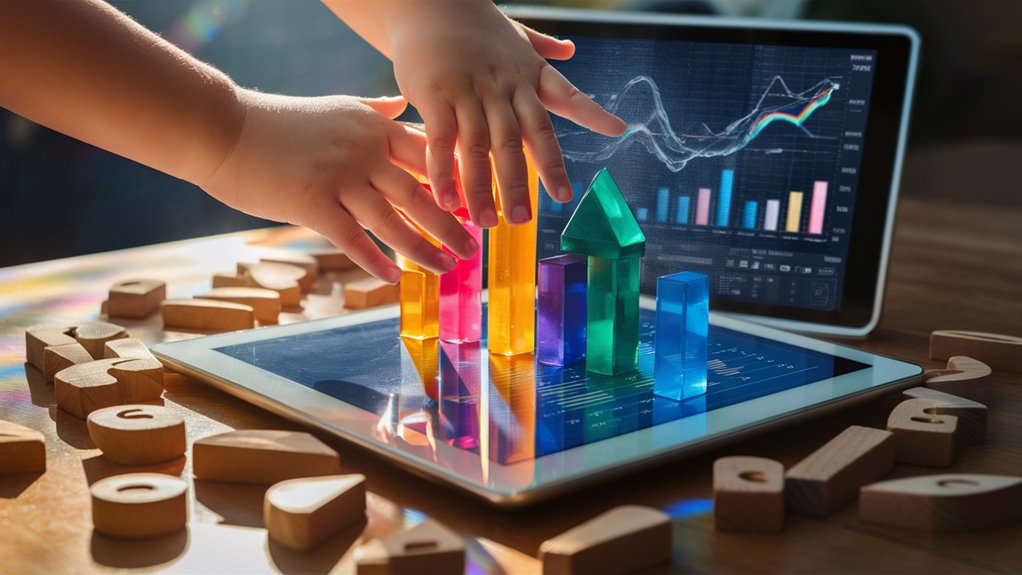
From Numbers to Narratives: Transforming Data into Compelling Stories
Understanding Data Storytelling
*Data storytelling* represents the critical bridge between raw analytics and actionable insights.
*Effective data narratives* combine statistical precision with contextual intelligence, enabling organizations to derive meaningful conclusions from complex datasets.
Key Components of Data Narrative Construction
*Statistical analysis* forms the foundation of compelling data stories.
Skilled analysts identify *meaningful patterns* and *correlations* within datasets, mapping these discoveries to real-world implications.
By examining *key performance indicators* alongside contextual factors, professionals can construct narratives that illuminate both causation and correlation.
Advanced Data Interpretation Techniques
*Pattern recognition* and *trend analysis* serve as essential tools for transforming numbers into narratives. When 메이저놀이터 먹튀검증 evaluating *customer behavior metrics*, analysts must consider:
- *User journey touchpoints*
- *Conversion funnels*
- *Engagement patterns*
- *Decision triggers*
Creating Impact Through Data Visualization
*Visual storytelling* enhances data comprehension through:
- *Interactive dashboards*
- *Trend graphs*
- *Heat maps*
- *User flow diagrams*
FAQ: Data Storytelling Essentials
Q: What makes a data story effective?
A: Effective data stories combine accurate analysis, clear visualization, and relevant context to create actionable insights.
Q: How do you identify key trends in data?
A: Analyze patterns over time, look for correlations between variables, and use statistical tools to validate observations.
Q: What role does context play in data interpretation?
A: Context provides essential background for understanding why patterns exist and what they mean for business decisions.
Q: How can visualization enhance data storytelling?
A: Visual elements make complex data more accessible and help audiences quickly grasp key insights and relationships.
Q: What’re the best practices for presenting data narratives?
A: Focus on clear messaging, use appropriate visualizations, and connect findings to specific business outcomes.
Best Practices for Implementation
*Data narrative development* requires systematic approach:
- *Establish clear objectives*
- *Identify key metrics*
- *Analyze relationships*
- *Develop context*
- *Create compelling visualizations*
Measuring Success
Track the effectiveness of data narratives through:
- *Stakeholder engagement*
- *Decision implementation rates*
- *Business outcome improvements*
- *Knowledge retention*
Blending Science and Spontaneity
*Blending Science and Spontaneity in Data Analysis*
*The Art and Science of Data Analysis*
*Scientific rigor* and *creative exploration* form the essential pillars of modern data analysis.
The systematic integration of these seemingly opposing approaches enables analysts to unlock deeper insights while maintaining methodological integrity.
Through strategic combination of *structured analysis* and *spontaneous discovery*, researchers can maximize their analytical potential.
*Structured Foundation*
*Methodological excellence* begins with establishing clear analytical frameworks.
By implementing *rigorous protocols* and *documented procedures*, analysts create a reliable foundation for their research.
Key elements include:
- *Formal hypothesis development*
- *Statistical methodology planning*
- *Data validation protocols*
- *Documentation standards*
*Creative Exploration Phase*
The *exploratory analysis* phase introduces controlled creativity into the analytical process.
During this stage, researchers engage in:
- *Unconventional variable testing*
- *Interactive visualization experiments*
- *Pattern recognition*
- *Outlier investigation*
*Integration Strategies*
*Balancing methodology* with creativity requires deliberate implementation strategies.
Successful integration involves:
- *Dedicated exploration time*
- *Separate documentation tracks*
- *Systematic validation*
- *Cross-verification protocols*
*Frequently Asked Questions*
Q: How can analysts maintain scientific rigor while exploring creative approaches?
A: By maintaining separate documentation for structured and exploratory analyses while cross-validating findings.
Q: What’re the key benefits of combining structured and creative analysis?
A: This approach reveals hidden patterns and relationships while ensuring methodological validity.
Q: How should analysts allocate time between structured and creative exploration?
A: Dedicate specific timeframes for each approach, typically favoring structured analysis (70%) over exploration (30%).
Q: What tools support both scientific and creative analysis?
A: Modern data visualization platforms, statistical software with exploratory features, and interactive notebooks.
Q: How can teams implement this dual approach effectively?
A: Through clear documentation protocols, designated exploration periods, and structured validation processes.
*Optimization and Implementation*
Success in *data analysis* requires strategic implementation of both *systematic methods* and *creative approaches*.
This balanced methodology enables analysts to:
- *Discover unexpected insights*
- *Maintain scientific credibility*
- *Document findings effectively*
- *Validate discoveries systematically*
Through careful integration of structure and spontaneity, analysts can achieve superior results while advancing the field of data science.
Making Space for Discovery
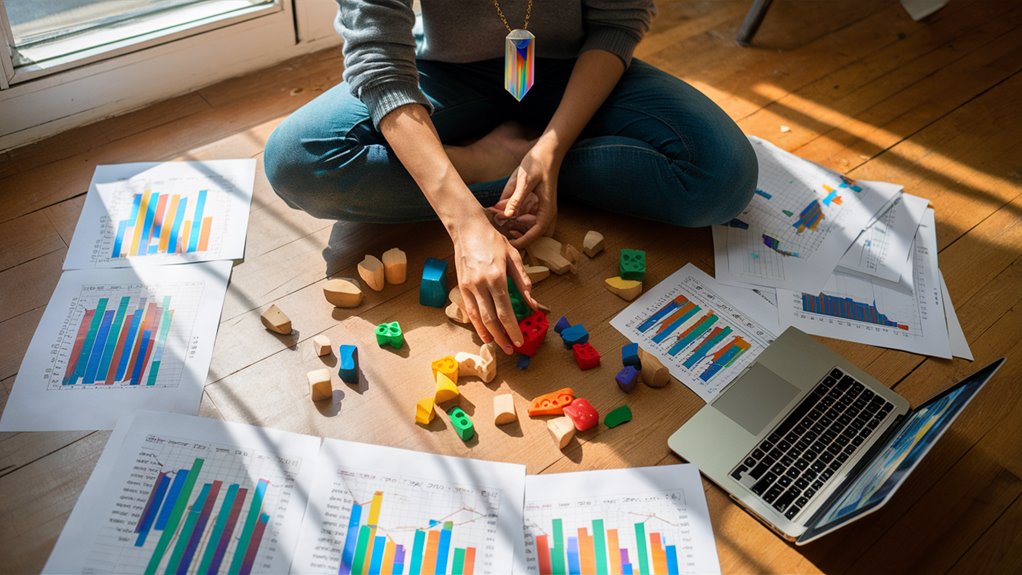
*Making Space for Discovery: A Guide to Data Analysis Innovation*
*Creating an Environment for Analytical Breakthroughs*
*Data analysis excellence* requires more than just technical skills – it demands dedicated space for genuine discovery. When analysts allocate specific time for *open-ended data exploration*, they often uncover critical patterns and relationships that structured methods alone might miss.
*Strategic preparation* begins with clearing your analytical pipeline of immediate deadlines and designating focused blocks for *experimental analysis*.
These discovery sessions enable testing of unconventional variable combinations, alternative visualization approaches, and challenge standard analytical assumptions.
Implementing a *systematic documentation process* captures both successful insights and valuable learning opportunities from unsuccessful attempts.
*Balancing Structure and Flexibility*
The most impactful discoveries emerge from maintaining equilibrium between *structured investigation* and *creative analysis*.
Using statistical foundations as a framework while remaining open to unexpected data relationships creates opportunities for breakthrough insights. When unusual patterns emerge, thorough investigation often reveals valuable connections, particularly when combining datasets in innovative ways.
*Frequently Asked Questions*
*How can analysts create effective discovery spaces?*
- Designate specific time blocks for exploration
- Remove immediate deadline pressure
- Create a systematic documentation process
- Maintain a distraction-free environment
*What tools support analytical discovery?*
- Data visualization software
- Statistical analysis programs
- Documentation platforms
- Collaboration tools
*How often should discovery sessions be scheduled?*
- Regular weekly blocks
- Project milestone reviews
- After significant data updates
- During strategic planning phases
*What are the key benefits of discovery space?*
- 혼란스러운 베팅장에서의 플레이
- Innovative analytical approaches
- Deeper dataset understanding
- Improved research outcomes
*How can teams balance structure and flexibility?*
- Set clear exploration parameters
- Allow for methodology adaptation
- Document unexpected findings
- Integrate discoveries into formal analysis
Remember: *Intentional discovery space* leads to enhanced analytical depth and originality, ultimately driving more valuable insights and innovative solutions in data analysis.