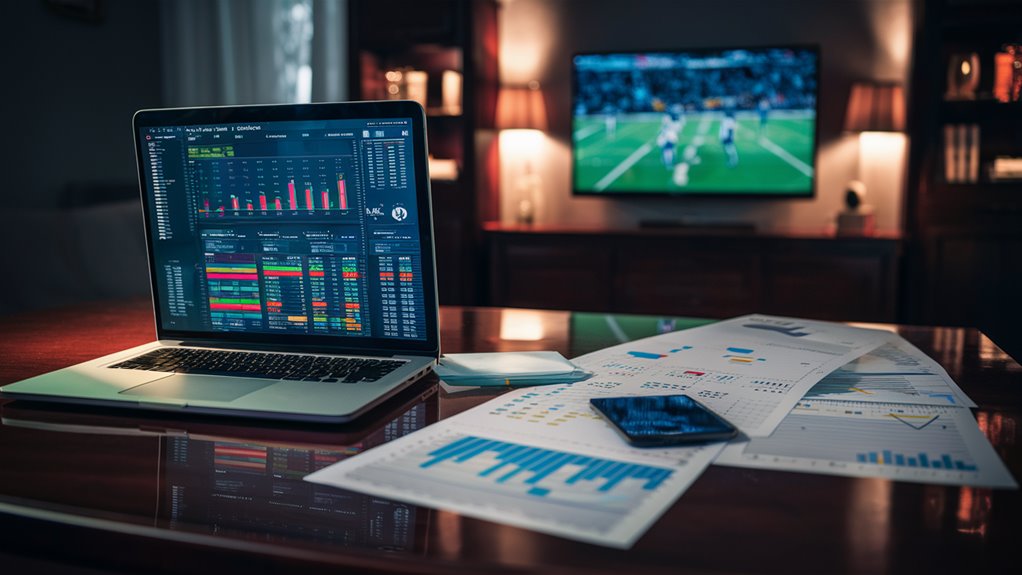
How to Use Data in Sports Betting
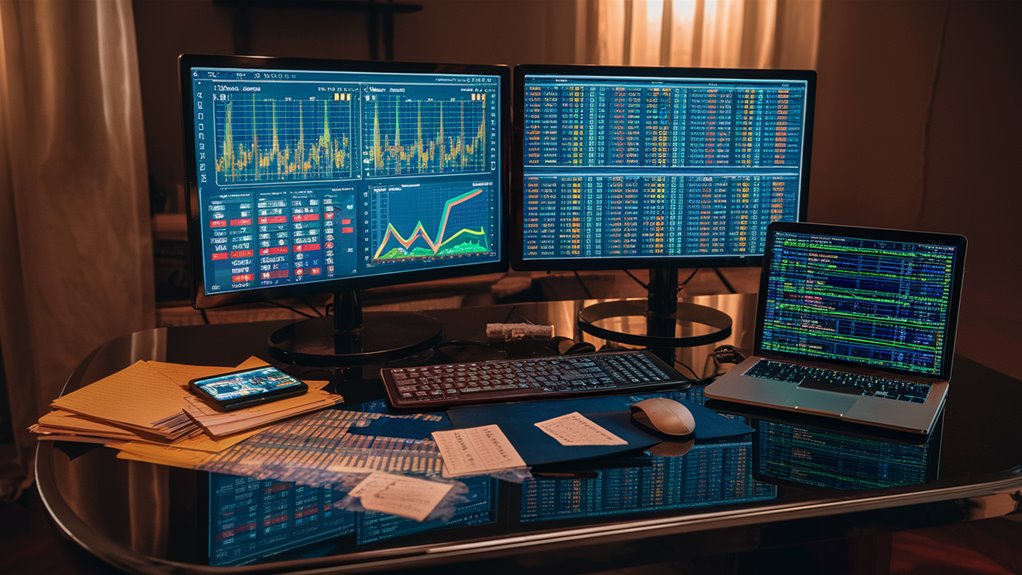
Making a Bet Plan Based on Data
Using sports betting data well means knowing many kinds of data to stay ahead. Looking at stats is key, and focuses on must-know numbers like win-loss data, score gaps, and past bet trends. Having good data sources and sports apps helps you track things as they happen and make smart choices. 여기서 안전성 확인하기
Putting Advanced Analytics to Work
Forecasting is at the heart of a top betting method, using things like regression and learning from data. These tools find great bet chances by looking deep into past and present data. Python and R are strong tools for making complex models that can spot when the market isn’t spot on.
Managing Your Money and Risks
Using firm money rules through the Kelly Rule tunes bet sizes and keeps money safe. Rules on how much to bet help keep your betting smart while aiming for good returns. Mixing data tools and risk in one full bet system uses data for smart plans.
Key Tech Parts:
- Stats tracking setups
- Forecasting designs
- Data learning uses
- Risk rules
- Seeing data clearly tools
Knowing Basic Stats
Getting the Key Stats in Sports Betting
Basic Stats
Looking at stats is vital for strong sports betting plans.
The main numbers look at performance info, past trends, and signs that bet well.
Win-Loss Study
Win-loss data gives key base info with two main checks: straight-up results and versus the odds.
Checking these shows good bet worth and market rightness. How real results match bet market hopes often shows good chances.
Score Numbers and Gaps
Score gaps are big in guessing right when checked right. Key parts include:
- Average score made
- Common point totals
- Point gap trends
- Home/away score changes
Stats Matter and Sample Size
Needs for samples ask for at least 20-30 games for first checks, with 50+ games being best for trust in stats.
Ranging stats help tell:
- How steady results are
- Result changes
- Spotting odd results
- Trust in trends
Top Performance Numbers
Pythagorean guess mixes with smart efficiency numbers to make prediction models.
Momentum signs track recent game trends through:
- Last 5-10 game results
- Scoring skill trends
- Schedule strength effects
- Home/away game splits
These top metrics shape full analytic designs for spotting key betting chances.
Kinds of Forecasting Models
Different Forecasting Ways in Sports Betting Data
Getting to Know Main Forecasting Types
Big changes in sports betting data make predictive models key, offering sharp ways to say what might happen and find bet spots.
Four main model kinds lead: regression models, learning from data, simulation models, and rating setups.
Regression-Based Guess Methods
Models based on regression, like logistic and linear, are core tools in checking links between key facts. These models are good at:
- Giving win chances
- Making point spreads
- Finding links between performance numbers
- Guessing score gaps
Advanced Data Learning Use
Data learning has changed how to analyze sports bets by dealing with complex patterns. Key uses include:
- Neural setups for deep pattern seeing
- Random forest models for checking many factors
- Gradient boosting for better guess skill
- Support vector machines for sorting
Guessing Based on Simulations
Things like Monte Carlo simulations and other models let us see many possible cases by dealing with:
- Player stats
- Where games are played
- Team matchups
- Past results
- Game-specific facts
Ranking Systems and Power Lists
Ranking setups give a system to sort teams through:
- Elo score math
- Power ranking ways
- Team strength checks
- Based on performance lists
- Head-to-head tests
Top bet plans often mix many model kinds, using each one’s strong points while fixing weak spots.
Key Performance Signs
KPIs in Sports Betting Data
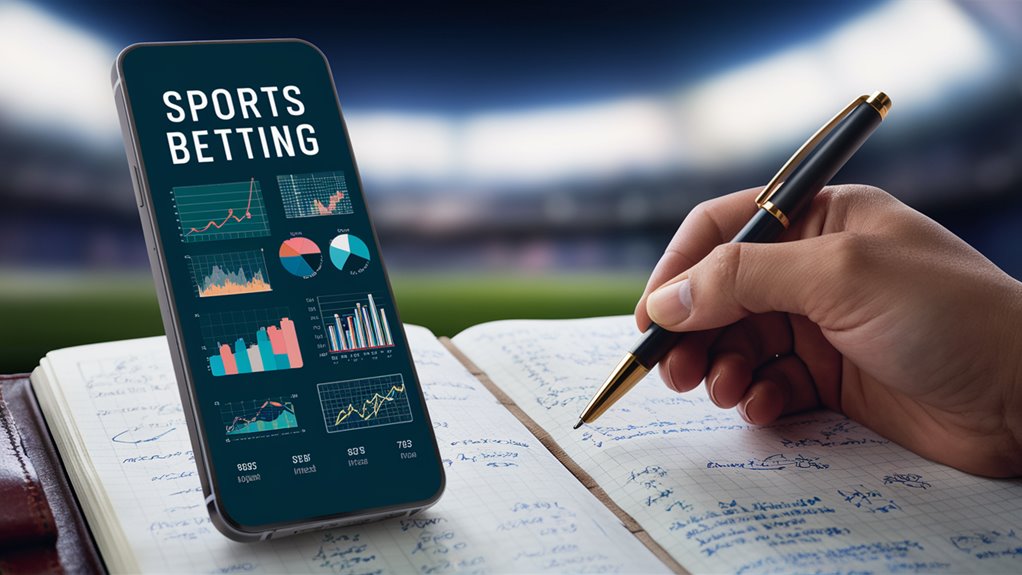
Main Bet Performance Numbers
Key numbers set the solid base for sports betting data by keeping track of important betting results.
Needed betting signs cover Return on Investment (ROI), win rate, usual odds, closing line value (CLV), and bet unit steadiness.
Main Performance Numbers
ROI tells how profitable, using net gains divided by total bet amount.
Win rate shows how often you win, while usual odds show your risk plan and if your way works.
CLV is key, checking caught worth against final odds and showing skill in seeing good market moves.
Data Tools and Seeing Markets
Must-Have Data Tools for Sports Info
Data Now and Past Records
Deep sports info needs lots of data and real-time feeds.
Sportradar and STATS give needed live sports data, and ESPN’s API and Sports Reference give lots of past stats needed for deep pattern seeing and trend checks. These are key to modern sports data work.
Stats Tools and Coding
Data use and predictive designs get better with strong code languages like R and Python.
Important tools like pandas and scikit-learn let us do deep stat analysis.
BetLab Pro and Sports Insights give special features for tracking market moves and finding good bet spots across different bookies.
Seeing Data and Checking Markets
Microsoft Excel is key for quick sums and simple seeing needs, while Power BI lets you make sharp analytic boards.
OddsPortal setup lets you watch markets fully, and auto web scrapers gather data from many places well.
The Action Network boosts analysis with its own algos and group tracking, finishing a strong setup for data-driven choices.
Key Tech Parts
- Data now feeds
- Past record places
- Predictive tool uses
- Market look setups
- Seeing software
- Auto data get systems
These linked tools build a full analytic setup that turns raw data into clear steps for better performance checks and choice making.
Risk Rules
Full Risk Rules for Sports Info
Main Risk Ideas
Smart risk rules are the base for good sports betting info, asking for a planned way to keep money safe and size right. How to Avoid Online Gambling Pitfalls
The Kelly Rule gives a math way to tune bet sizes based on seen edge and money, with part Kelly uses (25-50%) suggested to keep changes low.
Money Plans
Using different money ways in many bet areas helps keep money stable, with single bets kept to 2-3% of total money.
Planned stop-loss levels set daily (5%) and monthly (15%) protect against long bad runs and stop choices based on feelings.
More Ways to Cut Risks
Ties in markets are key in checking risks, mainly when looking at related bet chances.
Auto systems watch how much you put in different leagues and bet kinds, setting off alerts when risk goes over set limits.
Monte Carlo model use lets us test bet plans well, checking possible bad cases. This data-backed risk setup keeps making money over time while guarding against bad market times.