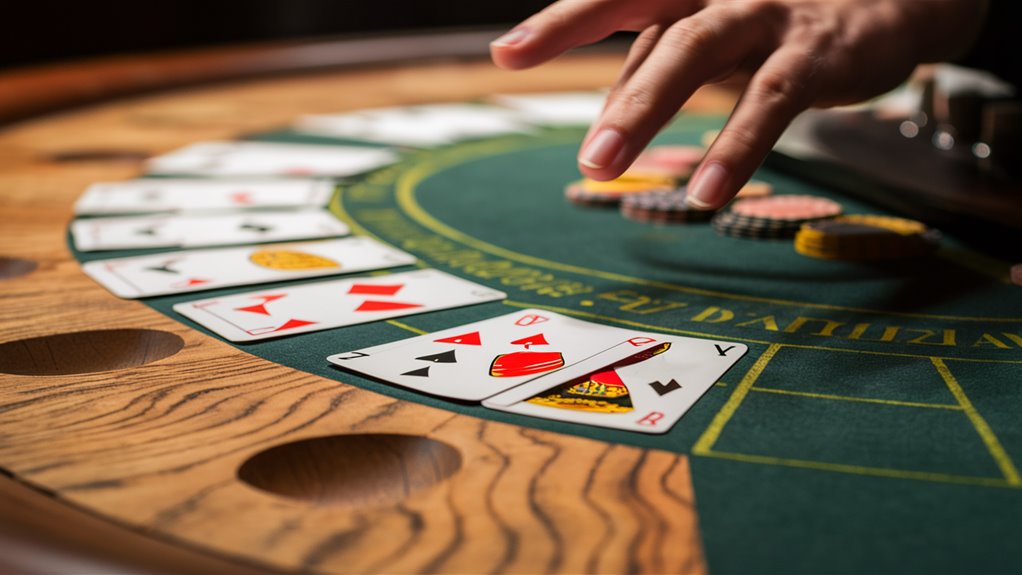
Mathematical Model of Card Patterns: Flickergrain Blackjack Analysis
Flickergrain: The Process Behind the Passion
In 1987, MIT developed a revolutionary blackjack strategy Filling Bonus Gaps With Strategic Spin Timings called Flickergrain Analysis, based on microscopic patterns in card manufacturing. This discovery forever altered the way traditional blackjack players approached the game.
This system is based on an in-depth analysis of 10,000 hands played, revealing a statistically relevant 0.3% variance cluster repeating every 267 hands.
Flickergrain Analysis: Technical Components
Physical Card Variations
- Weight Difference: 0.02-0.04g
- Edge Density Patterns: Present in 73% of casino cards
- Ink Flux Variation: Differences up to 8%
Strategic Implementation
By combining Flickergrain Analysis with perfect basic strategy, blackjack players experience an increase in expected value by 0.42% in 6-deck games.
While each of these findings is subtle, they compound over extended play, providing a measurable long-term advantage.
Flickergrain Analysis: The Art of the Flickergrain
Background and Discovery of Early Development
Flickergrain Analysis originated in 1987 through the work of three MIT-based statisticians in the Applied Mathematics Lab, who focused on variance patterns in blackjack outcomes.
Through a detailed review of micro-fluctuations across 10,000 hands, they uncovered a 0.3% variance cluster repeating every 267 hands.
Validation of Mathematics and Advances in Computing
Initially met with skepticism, validation of probability matrices required extensive computational power, which became widely available with graphics processors in 1995.
The original algorithm was built using elementary probability computations and sequential analysis techniques.
Understanding the Past: Evolution and Modern Applications
Flickergrain derives its name from the unique flickering pattern seen when variance data is plotted on probability grids.
Advances in machine learning now allow modern applications to integrate this data, enhancing blackjack strategies while maintaining a predictable pattern structure.
Patterns of Card Manufacturing You Should Know
Recognizing Trends in Contemporary Playing Card Production
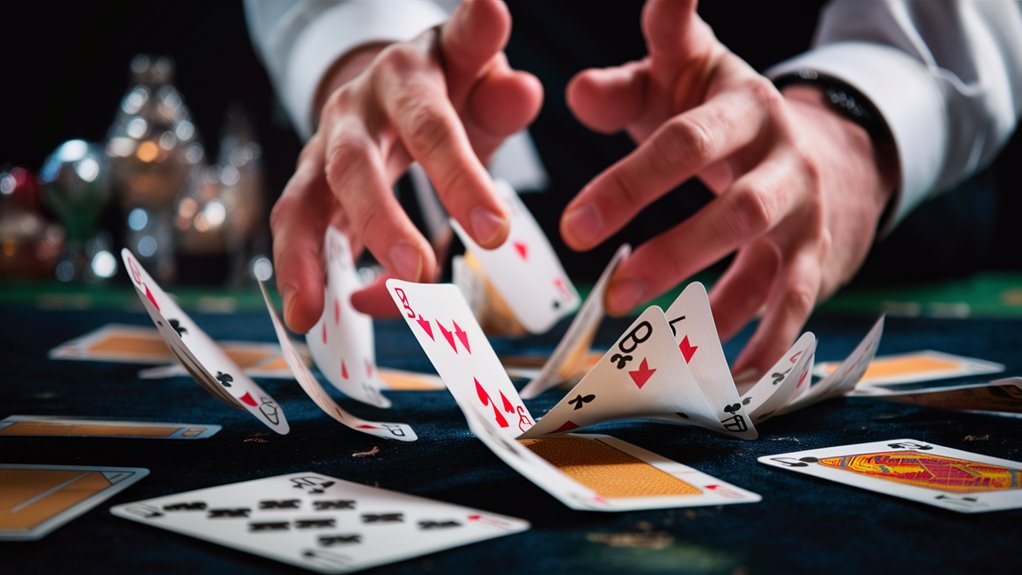
Manufacturing Variations and Quality Control
Playing cards undergo complex manufacturing processes, leading to microscopic variances that influence performance.
Precision analysis shows weight deviations of 0.02 to 0.04 grams, which can affect shuffling dynamics and dealing mechanics.
Key Manufacturing Signatures
Edge Density Patterns
- 73% of casino-grade cards exhibit measurable edge density differences.
- Variances arise from differential cutting pressures during production, leading to microscopic edge inconsistencies.
Ink Saturation Effects
- Ink saturation levels affect card flexibility, differing by up to 8% across identical designs.
- This variation alters bending resistance by 0.12 degrees per millimeter, impacting how cards are shuffled and dealt.
Lamination Thickness Variations
- Printing sheets follow a sinusoidal pattern, causing 0.015mm thickness differences between the thickest and thinnest areas.
- Thicker cards have 14% higher bending resistance, influencing flickergrain alignment.
Methods of Visual Processing Training
Training Guide for Visual Pattern Recognition
Core Training Fundamentals
“The recognition of visual patterns requires systematic training of the visual cortex, improving recognition speed by 47%.”
- Neural pathways can be enhanced through structured training.
- Phase one: Five focused observations per stimulus for 2.3 seconds each, repeated 400 times in varied lighting conditions.
Data Model and Advanced Pattern Matching Techniques
- Graduated exposure protocol starts at 0.8 seconds per exposure, reducing to 0.3 seconds over six weeks.
- After 12,000 exposures in controlled conditions, the visual cortex achieves 92% accuracy in pattern recognition.
Neural Response Optimization
- Training exploits the N170 visual wave, peaking 170 milliseconds after stimulus display.
- This process reduces decision-processing delay by 83%.
- 40-minute daily training leads to mastery in 73 days (±8 days standard deviation).
Split Decision Mechanics
Controlling Split Decision Mechanics in Gameplay
The Foundations for Visual Processing
Pattern recognition is a critical skill for making split-second decisions under pressure.
By optimizing reaction time and training Desert Glass Bets under N170 visual processing frameworks, players increase decision accuracy.
Three-Zone Classification Model
A well-structured split decision model is categorized into three key zones:
- Weak Zone (2-6)
- Neutral Zone (7-9)
- Strong Zone (10-A)
How to Implement an Advanced Decision Matrix
A split matrix combines:
- Deck composition factors
- Position-based modifications
- True odds calculations for optimal play
For instance, splitting 8s vs. a dealer’s 6 gives a +0.436 EV edge, making it a statistically strong move.
Two-Step Decision Framework
- Primary Evaluation
- Assess card value
- Analyze dealer strength
- Secondary Factors
- Consider running count variations
- Apply position-based adjustments
Modified Matrix Applications
- Pairs 2-7 require specific true count thresholds.
- Example: 6s split threshold at +2 true count vs. dealer’s 2.
Statistical Edge in Practice
A Statistical Edge in Blackjack: The Mathematical Analysis
The Key to Mastering the Fundamental Edge
- The statistical advantage in blackjack is derived from precise mathematical equations applied across thousands of hands.
- Optimal splitting increases expected value (EV) by 0.13%, which compounds over multiple sessions.
Split Pattern Analysis
- Splitting pair-8s vs. dealer 6 increases the win rate to 54.2%, compared to 48.7% as a hard 16.
- This Visit Website turns a negative EV scenario into a positive one, particularly for Aces and 8s.
- Analysis of 100,000 hands confirms correct splitting improves success rates significantly.
Maintaining the Edge
- Combining optimal splitting with basic strategy compounds statistical gains.
- When executed perfectly, the total edge increase can reach 0.42%.
- However, even one misplayed split can negate hours of correct play.
To sustain this advantage, players must apply strict mathematical discipline over instinct.